Lecture 13 Generative Models Stanford University School Of
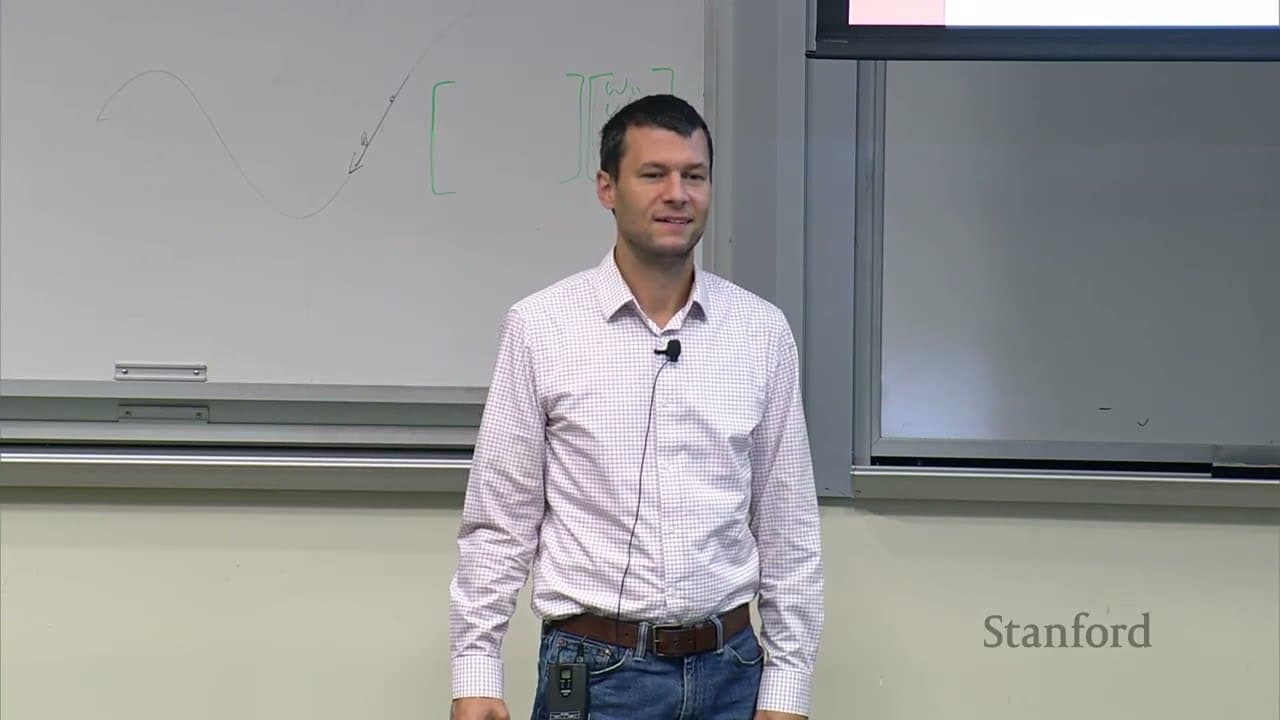
Stanford Cs236 Deep Generative Models I 2023 I Lecture 13 Score In lecture 13 we move beyond supervised learning, and discuss generative modeling as a form of unsupervised learning. we cover the autoregressive pixelrnn an. Lecture 13 20 may 12, 2022. fully visible belief network (fvbn) use chain rule to decompose likelihood of an image x into product of 1 d distributions: explicit density model. likelihood of image x probability of i’th pixel value given all previous pixels. then maximize likelihood of training data. fei fei li, jiajun wu, ruohan gao.

Lecture 13 Generative Models Stanford University School Of In this course, we will study the probabilistic foundations and learning algorithms for deep generative models, including variational autoencoders, generative adversarial networks, autoregressive models, normalizing flow models, energy based models, and score based models. the course will also discuss application areas that have benefitted from. Deep generative models. $1,750.00. course materials are available for 90 days after the course ends. course materials will be available through your mystanfordconnection account on the first day of the course at noon pacific time. a course syllabus and invitation to an optional orientation webinar will be sent 10 14 days prior to the course start. Explore score based models in this lecture from stanford university's cs236: deep generative models course. delve into the intricacies of these powerful generative techniques as associate professor stefano ermon guides you through key concepts and applications. Quantitative evaluation of generative models is a challenging task for downstream applications, one can rely on application speci c metrics for unsupervised evaluation, metrics can signi cantly vary based on end goal: density estimation, sampling, latent representations stefano ermon, aditya grover (ai lab) deep generative models lecture 13 21 21.
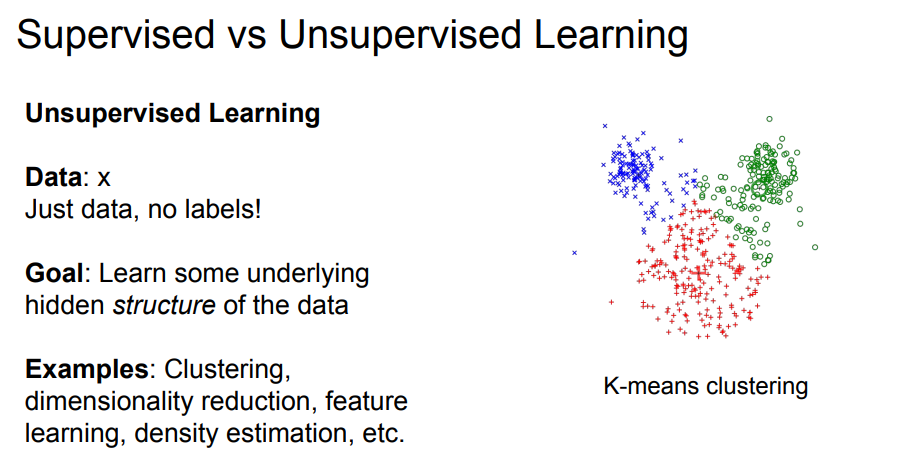
Cs231n Lecture 13 Generative Models Explore score based models in this lecture from stanford university's cs236: deep generative models course. delve into the intricacies of these powerful generative techniques as associate professor stefano ermon guides you through key concepts and applications. Quantitative evaluation of generative models is a challenging task for downstream applications, one can rely on application speci c metrics for unsupervised evaluation, metrics can signi cantly vary based on end goal: density estimation, sampling, latent representations stefano ermon, aditya grover (ai lab) deep generative models lecture 13 21 21. What are the pre requisites? basic knowledge about machine learning from at least one of: cs 221, 228, 229 or 230. basic knowledge of probabilities and calculus: students will work with computational and mathematical models. proficiency in a programming language: preferably python. Generative models are a key paradigm for probabilistic reasoning within graphical models and probabilistic programming languages. it is one of the exciting and rapidly evolving fields of statistical machine learning and artificial intelligence. recent advances in parameterizing generative models using deep neural networks, combined with.
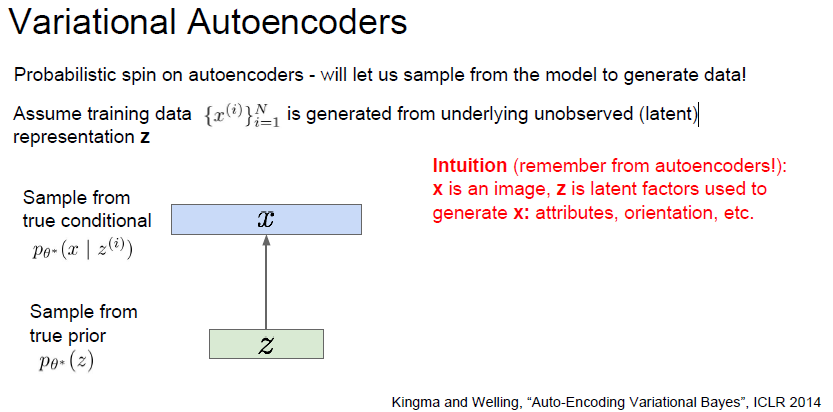
Cs231n Lecture 13 3 Generative Models Strutive07 лё лўњк ё What are the pre requisites? basic knowledge about machine learning from at least one of: cs 221, 228, 229 or 230. basic knowledge of probabilities and calculus: students will work with computational and mathematical models. proficiency in a programming language: preferably python. Generative models are a key paradigm for probabilistic reasoning within graphical models and probabilistic programming languages. it is one of the exciting and rapidly evolving fields of statistical machine learning and artificial intelligence. recent advances in parameterizing generative models using deep neural networks, combined with.
Comments are closed.