Lecture 13
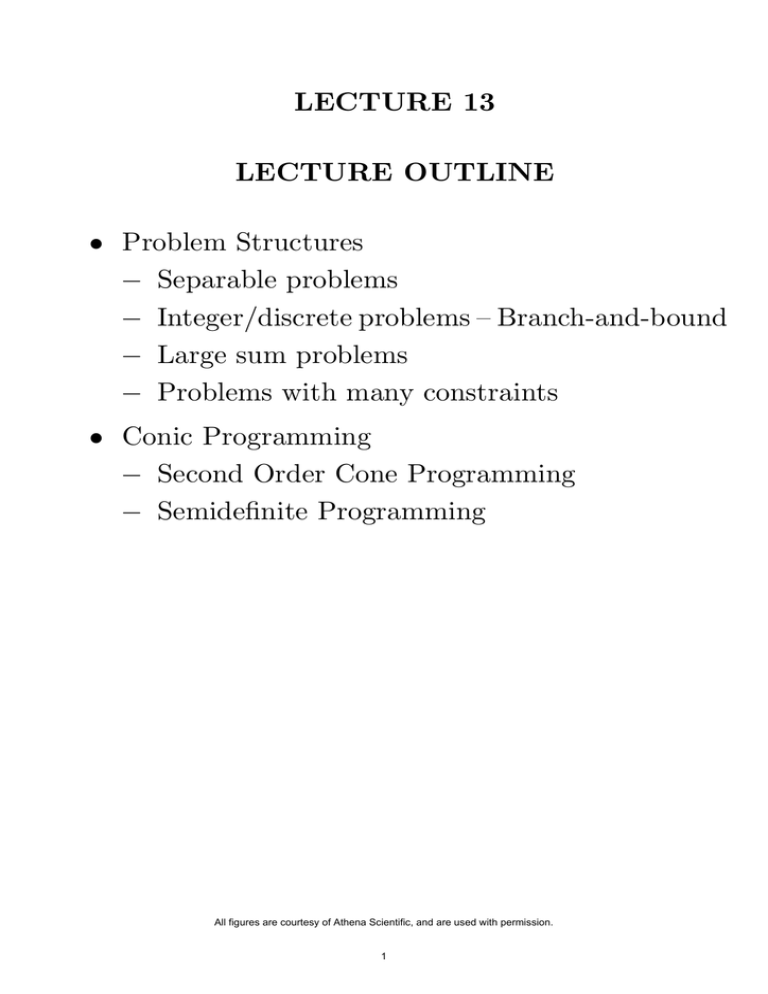
Lecture 13 Lecture Outline вђў в Mit 18.102 introduction to functional analysis, spring 2021instructor: dr. casey rodriguezview the complete course: ocw.mit.edu courses 18 102 introd. Lecture 13: search, part 3. tobias salz continues to discuss economic settings through the lens of search models. freely sharing knowledge with learners and educators around the world. learn more. mit opencourseware is a web based publication of virtually all mit course content. ocw is open and available to the world and is a permanent mit.
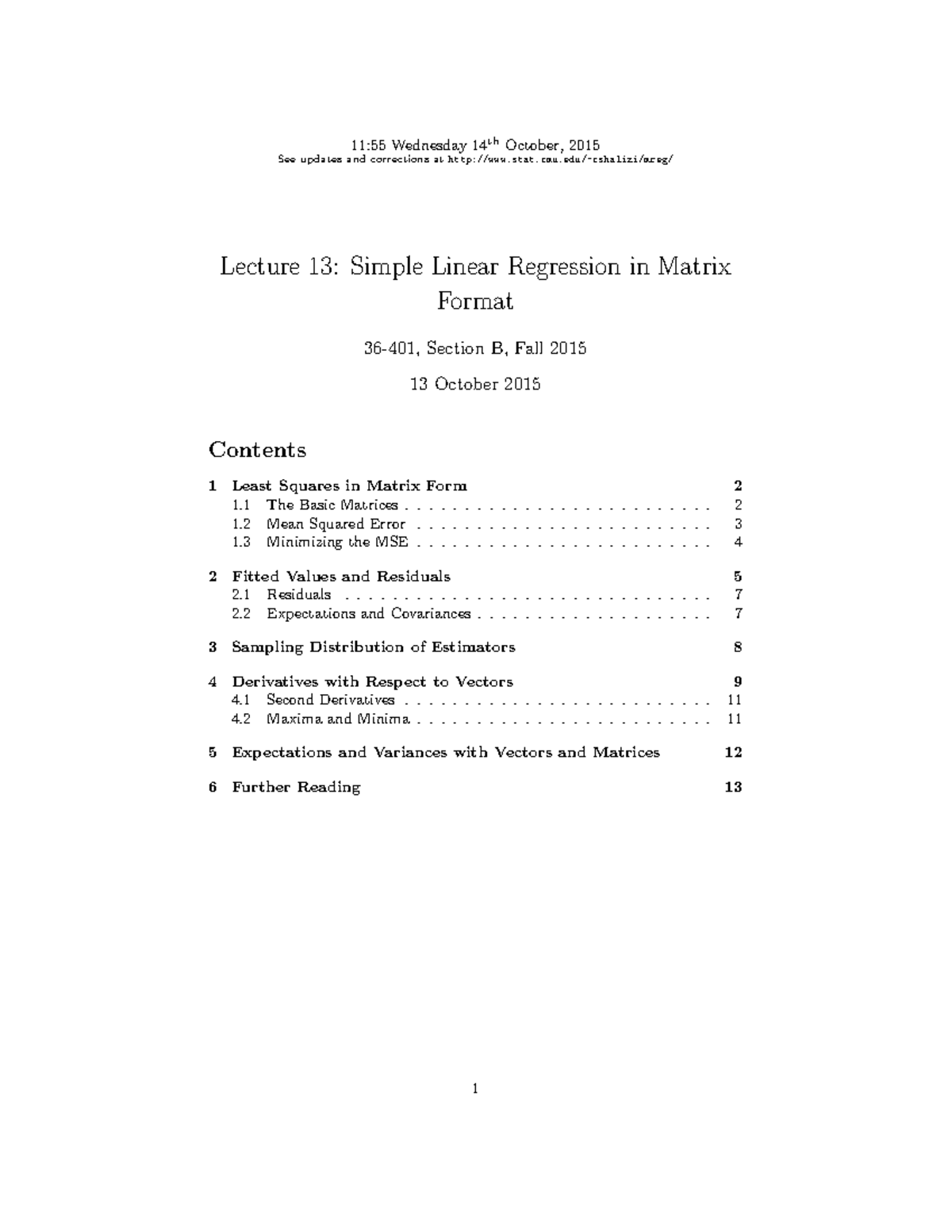
Lecture 13 Lecture Notes 13 11 55 Wednesday 14th October 2015 See Lecture 13: dijkstra this class discusses a fourth weighted single source shortest path algorithm: dijkstra’s algorithms. non negative edge weights are introduced. Lecture videos lecture 13: breadth first search (bfs) description: this lecture begins with a review of graphs and applications of graph search, discusses graph representations such as adjacency lists, and covers breadth first search. Lecture 13 introduces attention as a mechanism for deep networks to dynamically pay attention to different parts of their inputs. we see how recurrent networ. Mit 8.323 relativistic quantum field theory i, spring 2023instructor: hong liuview the complete course: ocw.mit.edu courses 8 323 relativistic quantu.
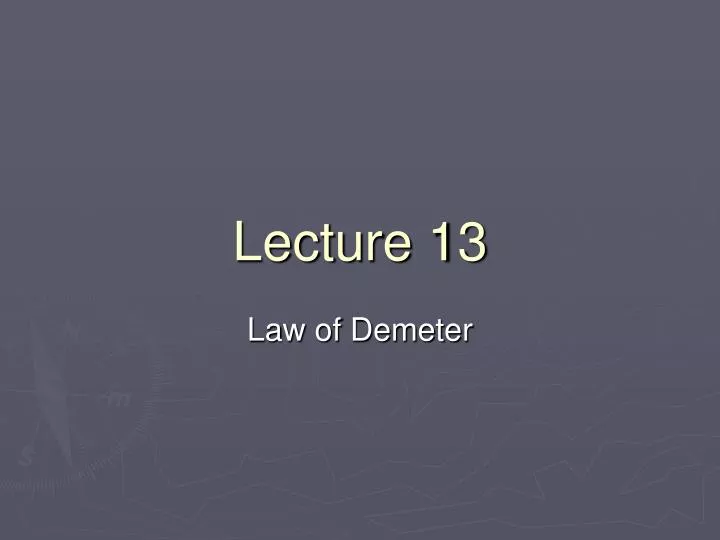
Ppt Lecture 13 Powerpoint Presentation Free Download Id 3973193 Lecture 13 introduces attention as a mechanism for deep networks to dynamically pay attention to different parts of their inputs. we see how recurrent networ. Mit 8.323 relativistic quantum field theory i, spring 2023instructor: hong liuview the complete course: ocw.mit.edu courses 8 323 relativistic quantu. Explore random walks and related concepts in this lecture from mit's computational thinking spring series. dive into julia programming language fundamentals before delving into pascal's triangle and its connection to convolutions. examine random walks as independent and identically distributed random variables, and learn how to represent them. Lecture 13 may 18, 2017 unsupervised learning data: x just data, no labels! goal: learn some underlying hidden structure of the data examples: clustering, dimensionality reduction, feature learning, density estimation, etc. 14 supervised vs unsupervised learning supervised learning data: (x, y) x is data, y is label goal: learn a function to.
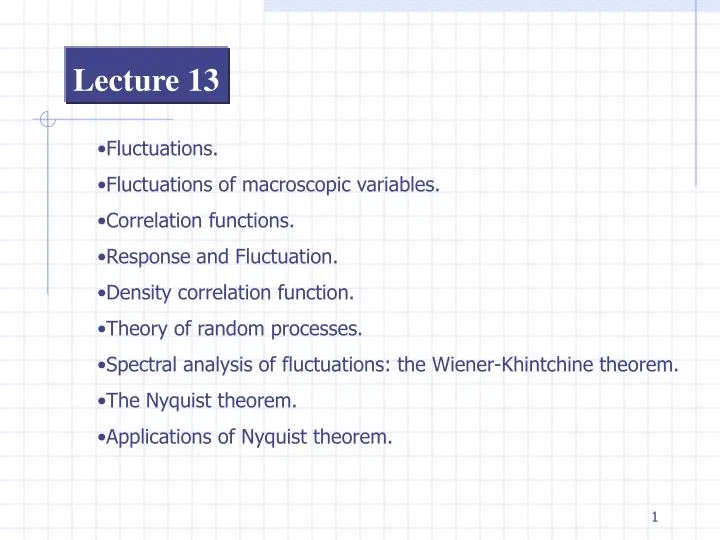
Ppt Lecture 13 Powerpoint Presentation Free Download Id 864363 Explore random walks and related concepts in this lecture from mit's computational thinking spring series. dive into julia programming language fundamentals before delving into pascal's triangle and its connection to convolutions. examine random walks as independent and identically distributed random variables, and learn how to represent them. Lecture 13 may 18, 2017 unsupervised learning data: x just data, no labels! goal: learn some underlying hidden structure of the data examples: clustering, dimensionality reduction, feature learning, density estimation, etc. 14 supervised vs unsupervised learning supervised learning data: (x, y) x is data, y is label goal: learn a function to.
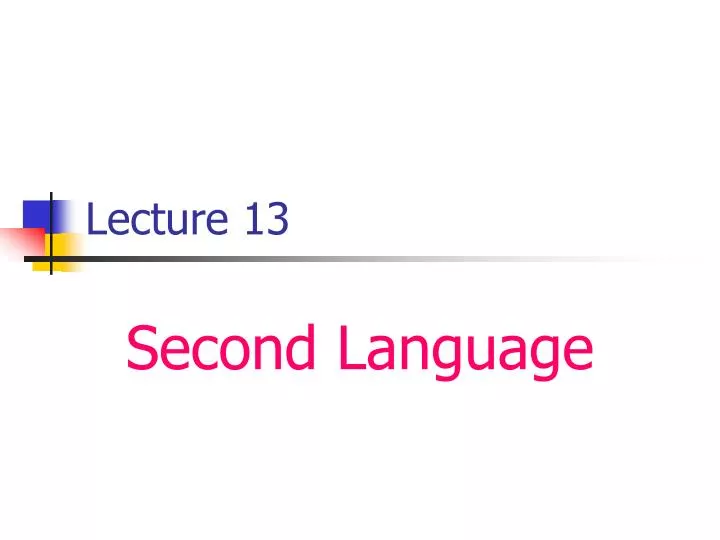
Ppt Lecture 13 Powerpoint Presentation Free Download Id 5175050
Comments are closed.